Banking supervision with the use of innovative statistical techniques
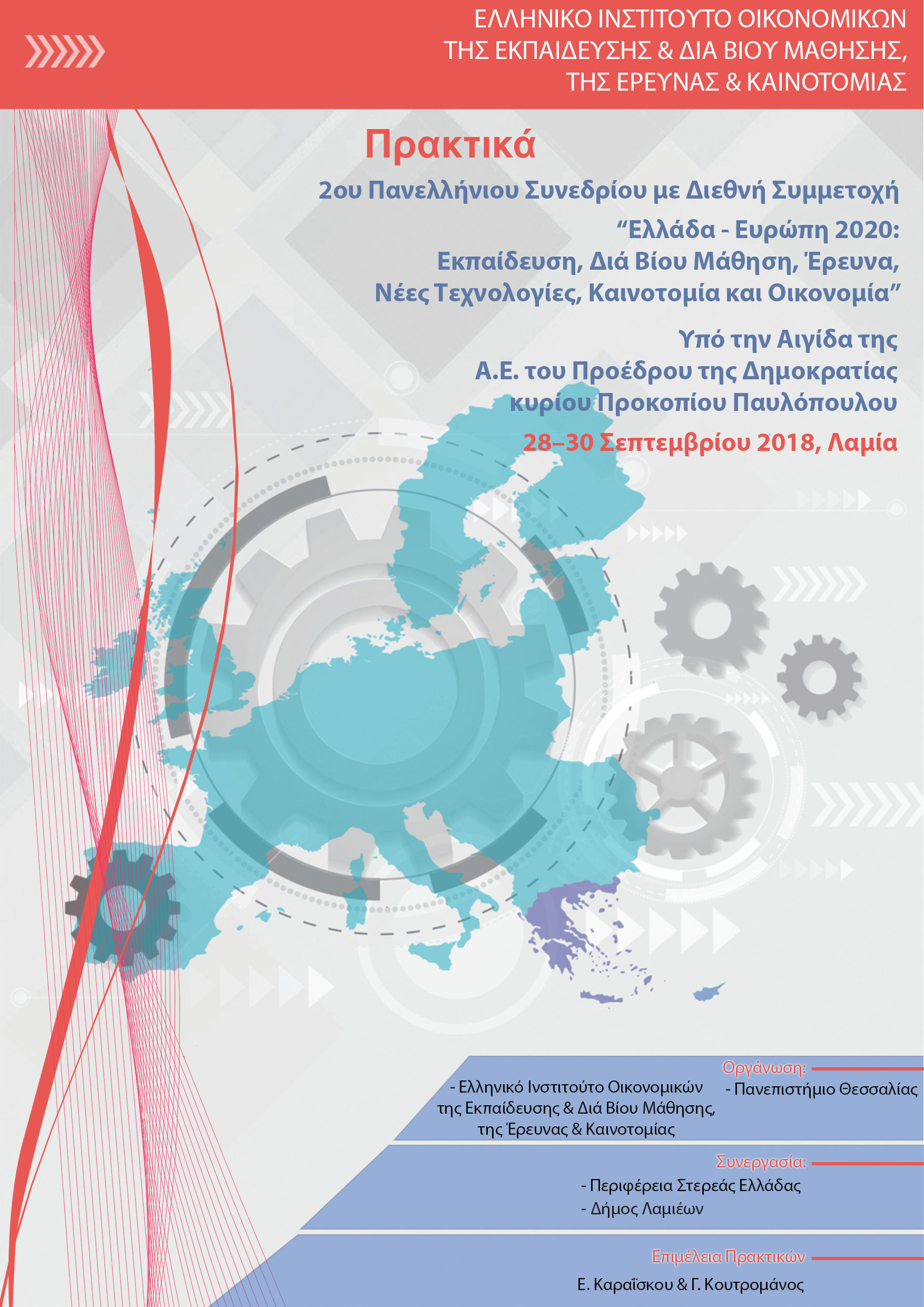
Abstract
Proactively monitoring and assessing the economic health of financial system has always been the cornerstone of supervisory authorities for supporting informed and timely decision making. Bank of Greece as the competent supervisory authority for the Greek banking system evaluates both the riskiness of banks on an individual level and the health of the financial system in total, from a macro prudential perspective. In accomplishing those targets, the Bank of Greece could make use –inter alia- of various statistical methods along with expert judgment. In this work, we employ a series of innovative modeling techniques in the prediction of individual bank insolvencies and generalized financial crises. Our empirical results indicate that innovative statistical techniques, i.e. Deep Learning and Machine Learning methodologies, have superior out of sample and out of time predictive performance in comparison to traditionally employed methods in finance, such as Logistic Regression, Classification Tress, and Linear Discriminant Analysis. In essence, we build an Early Warning System for bank insolvencies and another one for stock market crises, which could complement the assessments performed by micro-prudential and macroprudential authorities. In short, the holistic monitoring of the resilience of the financial system would steer decision making, via triggering the imposition of any necessary targeted corrective actions, leading vulnerable institutions back to viable business performance and the financial system back to balanced operation.
Article Details
- Section
- Εισηγήσεις